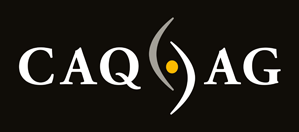
The R&D department at CAQ AG has researched the application of machine learning and artificial intelligence (AI) in order to identify non-valid measurement values. This knowledge today already comes to use in shape of a new type of data validation that facilitates the analysis of process deviations via the CAQ.Net software suite. Apart from a whole host of contemporary methods for analysing measurement data, CAQ.Net now allows the usage of an all-new method for identifying hidden irregularities and causes for deviations in any series of measurements.
Artificial Intelligence and the Fourth Industrial Revolution
Whether algorithms implemented by e-commerce companies, intelligent translation services in the web, image recognition or autonomous driving – seemingly omnipresent artificial intelligence and machine learning applications are steadily becoming integral parts of everyday life. It therefore comes as no surprise that these technologies are also rapidly moving into the field of quality assurance within the context of the Fourth Industrial Revolution.
Statistical Evaluation Methods
CAQ-systems today already provide a number of evaluation methods for analysing measurement values. Apart from fully automatically establishing the most suitable distribution model and calculating, evaluating, and displaying adequate parameters, CAQ-systems contain tools for process performance and capability evaluations. Applications for weak-point-analysis, identifying fault concentrations, batch tracking, and SPC complete the portfolio of contemporary CAQ-systems.
What are Valid Values?
All these tools, however, assume and are based on the fact that gathered measurement values are valid. But are they? Validity is the extent to which a measurement is well-founded and likely corresponds accurately to the real world. Hence, the validity of an assessment is the degree to which it measures what it is supposed to measure. This means that a measurement is valid if a gauge precisely measures and correctly displays the desired values and the inspector has followed his instructions properly. Reasons for non-valid measurement results may be uncalibrated gauges, faulty communication of inspection orders, or non-performance of inspections. Whereas these causes can be identified comparatively easily, identifying faulty or multiple data transmissions from measuring machines, deliberate measurement manipulations, or accidental measurement distortions is much more difficult.
Measurement Manipulations and Distortions
During the characterisation and identification of typical measurement manipulations and distortions, the Compact.Net software module analyses any type and length of measurement series. The values are hereby largely normed and classified in advanced. Following this, the software scans the measurements and checks whether e.g.:
- – The same value repeats itself across several measurements
- – The same distance repeats itself between two measurements
- – The values of a good sample are repeatedly copied
- – The value “scheme” of a good sample is repeatedly copied
The advantages of this intelligent measurement value analysis are many, as, apart from providing an additional level of data validation, it also facilitates a greater degree of process control and the possibility of visualizing manipulations and preventing false reporting in advance.
Quality Ensures Safety
Today companies in virtually all lines of industry need to embrace the advantages of digitalisation and use intelligent methods of information analysis in their daily processes. This particularly applies to the area of quality assurance. Apart from providing an increase in efficiency throughout the production workflow, the application of intelligent quality assurance methods also facilitates a greater safety of products. Being able to identify measurement distortions in a value series is not only about reducing potential fault costs or additional work, increasing customer satisfaction and advantages over the competition, but also about finding hidden errors in the production workflow, avoiding legal consequences, and quite frankly, preventing serious bodily harm being caused to human beings through faulty products.
More information on our website: https://www.caq.de/en/News/AI_in_Quality_Assurance