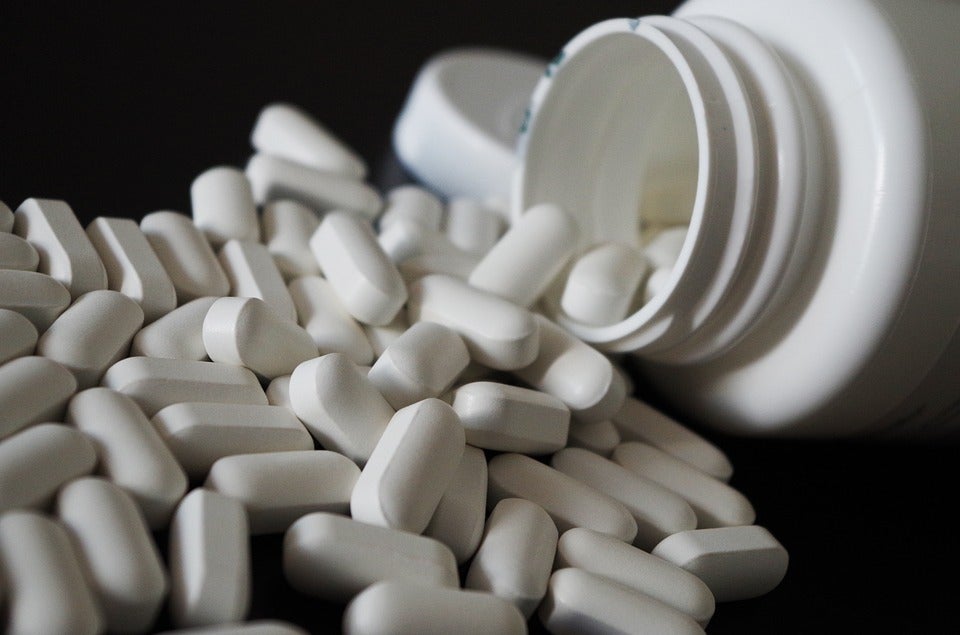
From bringing new drugs and therapies to market faster, to greater patient access to potentially life-saving treatment, AI could have a profound impact on the future of the pharmaceuticals industry.
Deploying effective AI-enhanced tools and deep learning techniques will provide a framework for companies to drive and optimise clinical trial processes, impacting engagement with customers, patients, suppliers and employees.
During a panel discussion at London Tech Week, speakers from major tech companies such as Microsoft, IBM and Google shared numerous ways they could make clinical trials intelligent using various forms of AI to improve data collection, thereby accelerating the development of new products.
Christina Busmalis, director of global life sciences at IBM Watson Health, said: “By applying broad AI, you can actually address the algorithms as the data changes.
“This will bring better time to market along with transparency, which is a key perspective of AI where we want to be able to see and understand many of the decisions made – it’s that ‘black box’ concept.
“At IBM, we call that explainable AI.”
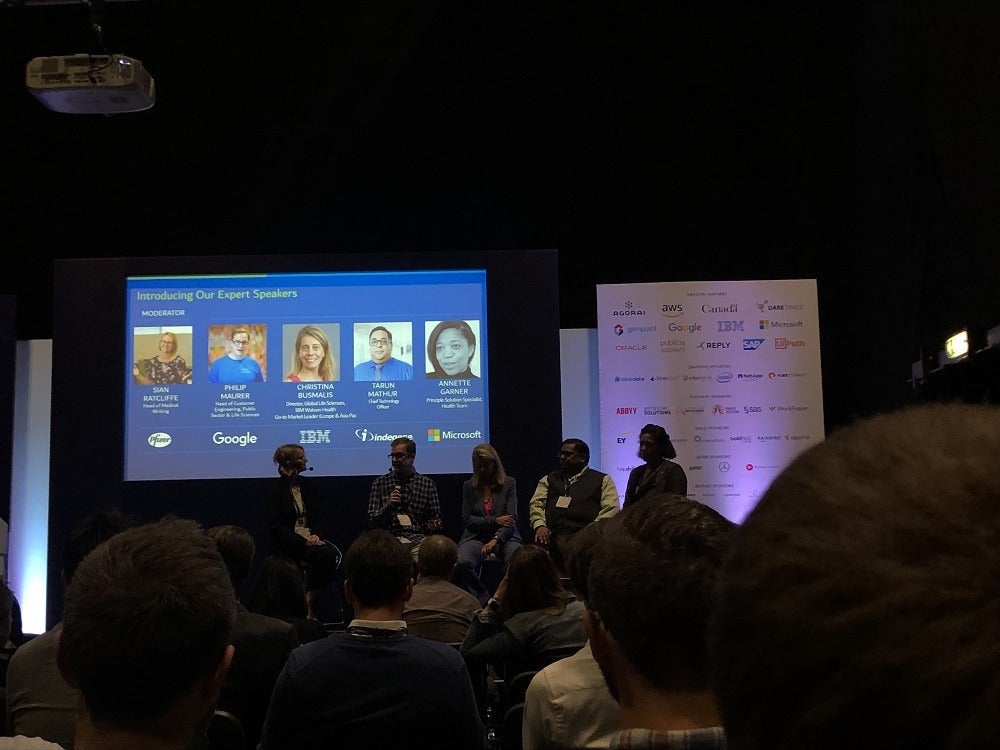
How AI is being successfully deployed broadly in the pharma commercial space
Interest in deploying AI has risen across many fields of business, with the healthcare industry already using it to enable machines to sense, comprehend, act and learn, so they can perform administrative and clinical functions.
Among the areas that could benefit from the use of AI, Ms Busmalis singled out commercial effectiveness, patient empowerment, digital therapeutics and physician medicine.
She added: “There are two examples I’ll give of AI tech that we developed in the area of diabetes, in which the underlying components of those is applying machine learning on real-world data.
“So one example is something we did with Roche, where we developed a predictive model using a machine learning algorithm to be able to predict patients at early risk of diabetes-related chronic kidney disease.”
The Roche-IBM predictive algorithm, which was trained using half a million electronic health records, outperformed published algorithms derived from clinical trial data in a one-to-one comparison, as well as in cohort studies.
“By understanding the patients’ stratification, you can actually target and apply that into commercial effectiveness,” said Ms Busmalis.
“Another area of diabetes is patient engagement. Diabetes is a disease that can be self-managed, with those suffering spending on average five hours a year with a healthcare practitioner.
“Glucose levels need to stay in their comfort zone, and can’t go too high or too low.
“And with Medtronic, we developed a global companion app called Sugar IQ, which actually helps patients manage their disease by providing recommendations on what to do and what to eat.”
The goal is for the technology to show people about their patterns and how lifestyle choices and medications impact their glucose ranges.
The app’s features include a smart food logging system, motivational insights, a glycaemic assistant and a data tracker to provide personalised recommendations.
Ms Busmalis added: “We show again that with machine learning, the tech is able to predict when someone will have a hyperglycaemic event two to four hours in advance, with 89% accuracy, allowing people to adjust their lifestyle to reduce the risk of that happening.”
How AI can be successful in a clinical setting for doctors and patients
Artificial intelligence is changing the way healthcare networks operate, with AI assistants helping physicians to make better clinical decisions.
Through relevant clinical questions, powerful AI techniques can unlock clinically relevant information hidden in the massive amounts of data, which in turn can assist clinical decision-making.
Philip Maurer, head of customer engineering, public sector and life sciences at Google, said: “Starting off with what we need to change, I think one of the key challenges in the clinical space is still data on probability.”
An example Mr Maurer provided to illustrate what can be achieved from successful data is an algorithm project he worked on with clinicians at the Moorfields Eye Hospital, in London, and DeepMind.
The team created an algorithm that can detect and recommend the correct course of treatment for more than 50 different types of eye illnesses.
It can also categorise them by the appropriate level of severity and explain how it arrives at each recommendation, which Mr Maura states is a “helpful approach, as even experienced clinicians make mistakes”.
He said: “Having that second opinion from a diagnostic algorithm is extremely helpful to make sure they get the best possible outcome.”
The research project addressed the “black box challenge” as the algorithm is based on two separate neural networks.
The first neural network gives the emission a three-dimensional-view of the OCT scan to display what the AI detected and what it “thinks is present”.
The second neural network takes the output from the first network and effectively provides a diagnostic, which is used on the patient by the clinician.
“Crucially, each of those data points that are presented by the AI also come with percentage points, so the clinician actually knows how confident the AI itself is,” said Mr Maurer.
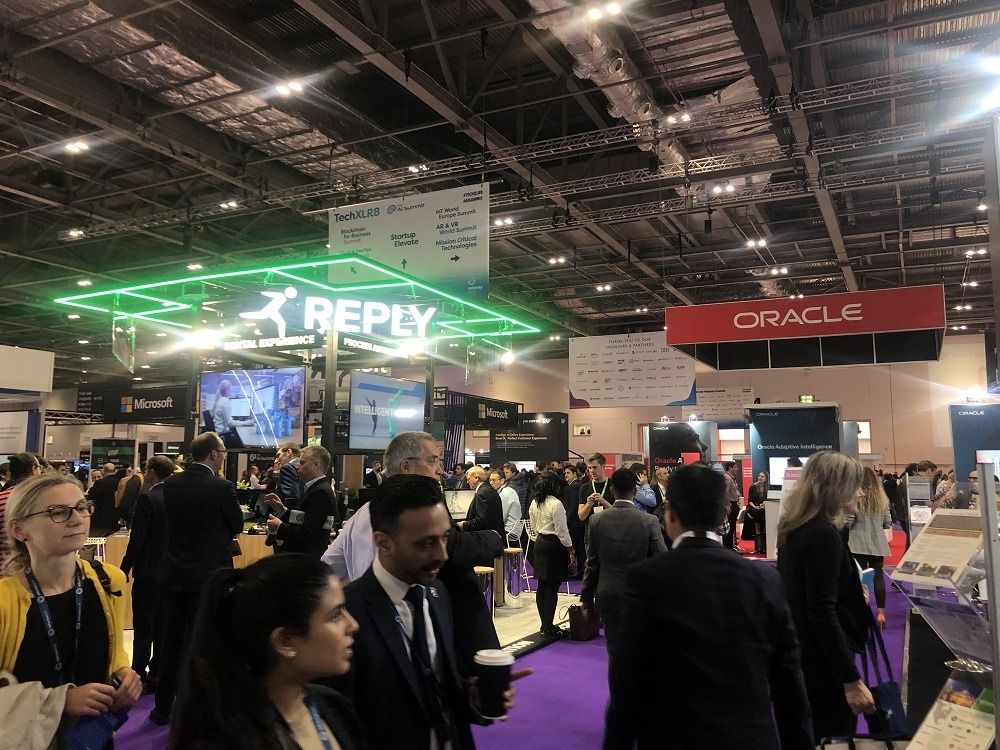
Clinical trials is a foundational pillar of the pharmaceutical drug discovery and marketing process.
A study published last year by the Massachusetts Institute of Technology has found that only 13.8% of drugs successfully pass clinical trials.
Furthermore, a company can expect to pay between $16m (£128m) and $2bn (£1.59bn) for any drug to complete the entire clinical trials process and get FDA approval.
Therefore, pharma businesses are using AI to increase the success rates of new drugs while decreasing operational costs at the same time.
Ms Busmalis said: “I think we all know in the industry, the holy grail was how could we get drugs to market faster and cheaper? It’s been that for decades and we’re still not there.
“With clinical trials, 80% of them don’t finish on time, 86% of them don’t have enough patients to recruit, and over 50% of them have to be amended. With every amendment, it costs more than half a million dollars.”
Ms Busmalis identifies the problem within the protocols, which she says can be very “sequential”.
She said: “We believe that AI can really change how you drive clinical trials and have a better clinical trial transformation.”
The protocols design area includes an inclusion and exclusion criteria – clinical end points in which AI can be used to help build the right protocol using historical information, and real-world data regarding what worked before – which can also be applied in patient recruitment.
She explained: “By having the right protocol and optimising it, you can move through clinical trials faster.
“You can also look at risk space monitoring and site selection to determine which sites are the right ones to use and which investigators are the right ones to work with.
“Again, machine learning should be applied in that area, understanding all those areas ahead of time will help drive clinical trials.”