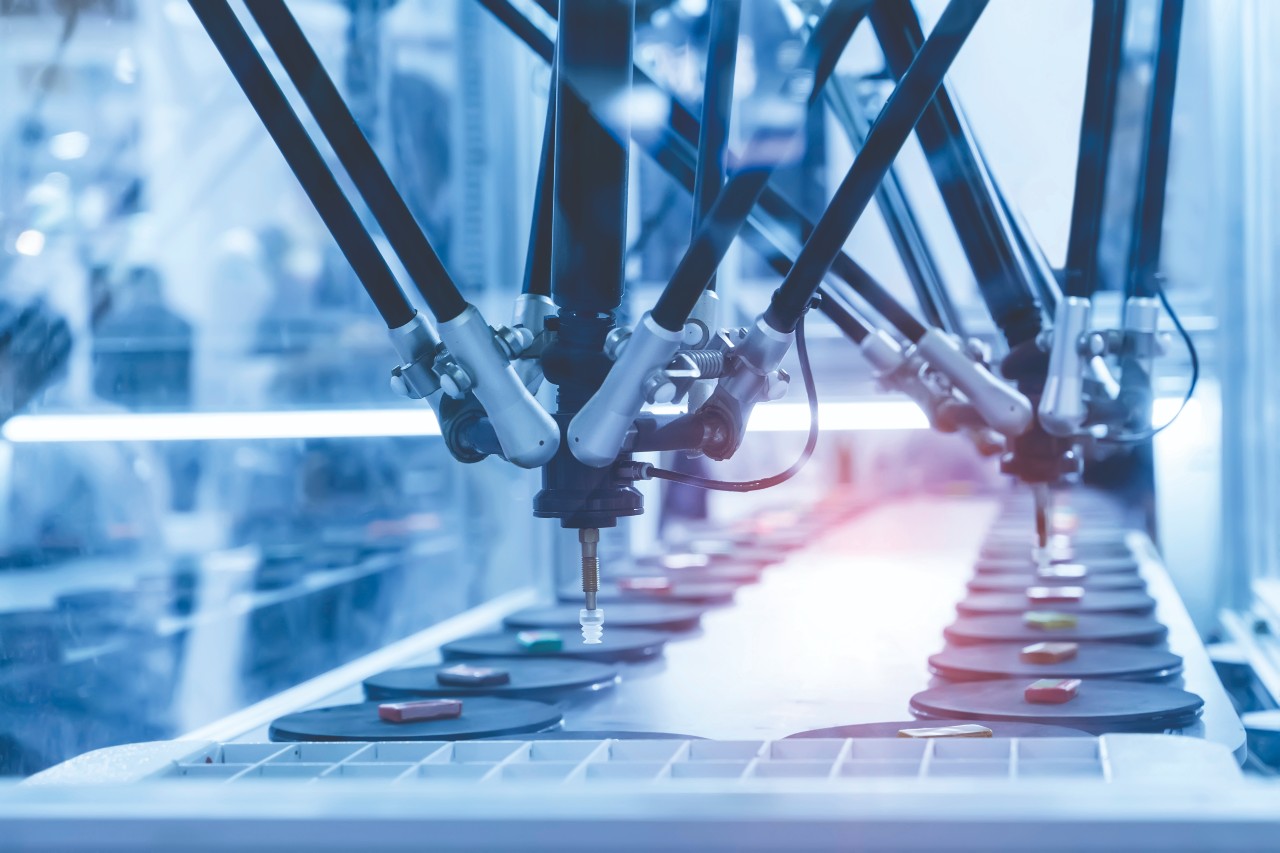
AI and machine learning have promised to transform medical device manufacturing for years – but, stymied by challenging data, it’s only recently being embraced by the biggest names in the industry. Andrea Valentino chats to Joe Corrigan, head of intelligent healthcare at Cambridge Consultants, about how new technology is making production oversight faster and more efficient.
For most of human history, medical devices were distinctly rugged affairs. Excavate a pit in Babylon or Sparta, or an Iron Age fort in the Welsh mountains, and you’ll understand – the rusting scalpels, the wobbly forceps, the metal hooks meant to hold up blood vessels and skin.
As late as the nineteenth century, in fact, many medical devices hadn’t really changed in thousands of years. They were made on an ad hoc basis, by doctors or small artisans, and sold to barber surgeons without any proper oversight or regulation.
A typically terrifying example comes from the volcanic ruins of Pompeii, where archaeologists recently found a rough metal speculum, probably used in childbirth.
Of course, this distinctly DIY approach has disappeared. For the past century or so, doctors have had access to a bewildering array of kit: from thermometers to hypodermic syringes, to ophthalmoscopes. That growth is reflected in the numbers.
As recently as the 1940s, the global medical device industry was worth less than $100bn. Now it’s in the trillions – and rising exponentially. That’s particularly true in the developing world.
According to one study, India’s sector now boasts over 4,000 medical technology start-ups, funded by $2.1bn in foreign direct investment. Across the Himalayas, meanwhile, China alone accounts for 20% of all global medical devices.
Yet if medical devices are now being pumped out in their billions – the EU, for its part, sells over 500,000 different models – the way they’re made hasn’t totally escaped the pattern of their ancient predecessors.
The artisanal workshops may have been replaced by assembly line factories, but many medical devices are still fundamentally built with humans at their heart.
Whether it’s the people on the shop floor searching for hairline cracks or those spending hundreds of hours in front of computer screens sifting through data to understand a problem, supply chains still rely on manual oversight to run smoothly.
Yet with the rise of sophisticated AI and machine learning technology, that could soon change – with enormous consequences for manufacturers and patients alike.
The potential of AI in medical device manufacturing
If you want to understand the future of machine learning in medical device manufacturing, you could do worse than tap Joe Corrigan. He began his career around 20 years ago, and has worked on everything from biomarkers for cardiovascular disease to imaging.
And, as the current head of intelligent healthcare at Cambridge Consultants, a market leader in developing new uses of AI in the medical field, it’s now his business to keep abreast of the latest research and applications. What he has to say is worth listening to – especially when it verges on revolutionary.
“The field of AI is moving forwards very quickly,” Corrigan says. This potential is certainly shadowed by the statistics. According to one report by PR Newswire, the healthcare AI market is projected to grow to $31.3bn by 2025, at a CAGR of 41.5%, with the biggest companies habitually securing hundreds of millions of dollars in funding from eager investors.
All the same, Corrigan cautions that, until recently anyway, device manufacturers struggled to use the extraordinary potential of AI in practice.
To understand what he means, think about a hypothetical manufacturing facility, making thousands of stethoscopes every hour. Most of the finished devices will be flawless – and some irredeemably damaged.
But what about in those ambiguous cases, when it’s unclear if a stethoscope has a fatal scratch or a simple knit line – or the light in the facility is just unusual and that makes it hard to decide?
In theory, an AI should be able to use machine learning and thousands of examples to make the right call, saving human operators hours of inspection time. Unfortunately, that’s not always been true.
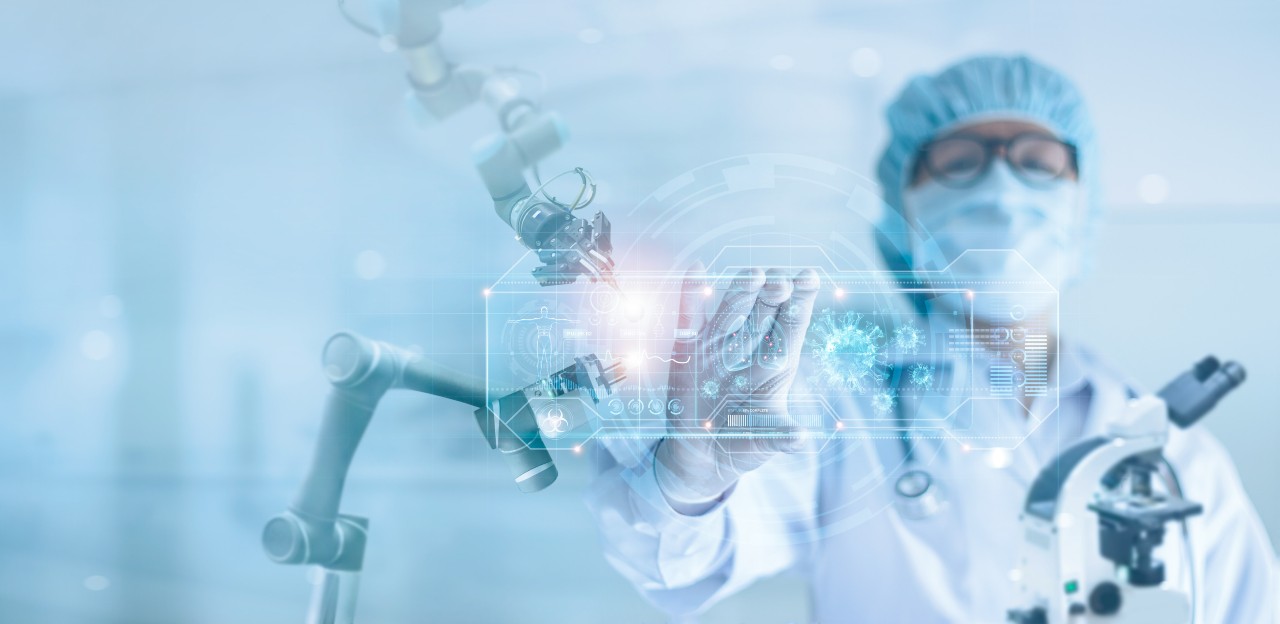
“The problem,” says Corrigan, “has been that training systems relied on either the expertise of the algorithm designer to identify the important features or for the training data to contain a complete set of flaws from which the algorithm can learn – but these may be very subtle, or sufficiently rare that they don’t appear in the training set.”
To put it another way, there have traditionally been too many variables for AI to fully understand the vagaries of the modern production line; unlike human operators, it can’t extrapolate to spot or solve issues for which it hasn’t specifically been trained.
Beyond the technology itself, meanwhile, manufacturing AI has had to deal with a host of other challenges. For one thing, it always has the potential of being overrun by hackers.
Given 88% of medical technology executives admit they’re unprepared for a breach, even though four in five suffered a cyberattack in the past five years, this is obviously a serious problem. Then there’s regulation.
Though Corrigan is reasonably bullish about working with officials – “for the most part, they’re OK with AI” – it’s clear that they’re also becoming more stringent.
That’s true on both sides of the Atlantic. In the EU, lawmakers recently proposed a certification regime for AI applications in medical technology.
The FDA’s new action plan makes similar noises and includes an expectation that manufacturers will keep it informed of any changes to their AI systems.
Using data as a device manufacturing strategy
Amid all these difficulties – and opportunities – how have Cambridge Consultants reacted? Arguably, their strategy can be boiled down to a single word: data. As Corrigan puts it: “We’ve been developing new systems that help operators to identify and map new types of flaws, building a huge catalogue of shared experience in a way that hasn’t been possible before.”
What does that look like in practice? Let’s return to our imaginary stethoscope factory. To stop the AI from crumbling in ambiguous cases – when it’s unclear if a device is ready to pass down the supply chain or needs to be binned – Cambridge Consultants would group the devices by feature.
Devices with one type of scratch go into one bracket, while those with another go into a second and so on. Eventually, there are enough examples for both human operators and their automated cousins to decide whether to pass or fail a particular machine.
The benefits of this approach are obvious. Rather than painstakingly checking quality by hand – which is anyway liable to mistakes – manufacturers can leave the legwork to the machines. That leaves more time to improve processes further up the supply chain, cutting waste and saving money.
At the same time, Cambridge Consultants has been developing systems to make its data analysis even better. Rather than rendering images of devices merely using 3D images with three colours, they now mix 3D video, hyperspectral imaging and lensless imaging.
Ultimately that makes it easier to spot defects and understand why they’re occurring. Nor are Corrigan and his team alone. In Germany, for example, Siemens is using AI to automatically check printed circuit boards. Given just 0.0008% of the devices were ‘not OK’, the scheme was evidently a success.
On an even grander scale, a group of ten major pharmaceutical companies, including Merck and Novartis, have teamed up to collectively train AI on larger datasets than any would be able to amass individually – making pharmaceutical manufacturing much faster for all of them. Of course, these changes can’t come about instantly.
As Corrigan explains, success instead requires close cooperation with stakeholders up and down the supply chain. “Most of our clients are very open about their goals,” he explains, “so we don’t just implement AI, but aim to support it and to help build teams within their organisation to allow it to grow.” The same is true of regulators.
With officials in Europe and the US more hawk-eyed than ever, Corrigan says that “finding the most efficient regulatory approach and explaining the validation approach to regulators requires a deep understanding of the algorithms being used, in order to convey how the validation leads to a safe and effective product.”
Cooperation for widespread appliction of AI to manufacturing
Corrigan suggests that similar AI and machine learning systems may soon be found up and down the supply chain, from factory floors all the way to clinics and operating theatres. He’s not the only one.
GE Healthcare and Philips both recently announced large investments in AI/ML, as did Medtronic, where Todd Morley, director of data science, “anticipate[s] widespread application of AI to manufacturing, including within our supply chain”.
At the other end of the healthcare system, there’s everything from embedding AI in inhalers to adding it to injectors.
Cambridge Consultants is even using technology to diagnose illnesses in real time. Rather than wasting time in pathology labs, in other words, doctors may soon be able to examine diseases immediately, saving patients potentially risky trips to the hospital.
We’ve obviously come a long way from those specula in Pompeii – and we won’t be the only intelligences deciding where we go next.
This article first appeared in Medical Device Developments Vol. 1 2021. The full publication can be viewed online here.