
The scientist behind a new AI tool for schizophrenia diagnosis says doctors will be able to improve decision-making by identifying the condition more precisely.
Dr Sunil Kalmady led a research team at the University of Alberta in Canada that created the EMPaSchiz software.
An abbreviation of ensemble algorithm with multiple parcellations for schizophrenia prediction, the technology has been developed to assist clinicians when performing MRI scans on a patient’s brain.
After spending seven years creating the software, a study published earlier this month found it had 87% accuracy in diagnosing the psychological illness.
Dr Kalmady told Compelo:“Diagnosis in psychiatry is largely based on subjective assessments by the psychiatrists, who agree that a more precise approach could improve clinical decision outcomes.
“However, medtech devices like functional MRI [fMRI] provide us with a way to obtain objective measurements of brain signals, and our machine-learned model EMPaSchiz accurately predicts schizophrenia using fMRI data.”
How EMPaSchiz is used for schizophrenia diagnosis
EMPaSchiz is an AI-based software that has so far examined brain scans from patients previously diagnosed with schizophrenia.
Researchers distinguished that EMPaSchiz is one of the first AI-powered learning tools trained exclusively on data from patients who had been diagnosed but not yet medicated to treat their illness.
As a result, the University of Alberta team believes the tech will be valuable in treating schizophrenia in its early stages.
Dr Kalmady said: “Subjects to be assessed by EMPaSchiz must undergo a five-minute fMRI scan of their brain in resting state because our model is trained based on brain activation and connectivity patterns.
“Next, the data is cleaned using standard neuroimaging software, which involves head motion correction and registration to standard brain template.
“Cleaned data can be then fed into EMPaSchiz, which can extract features related to regional activation and functional connectivity patterns, and then come up with the prediction whether the subject has schizophrenia.”
How EMPaSchiz beats current schizophrenia diagnosis methods
Dr Kalmady said the fact previous devices have been tested on patients on medication for schizophrenia skewered the results.
This is because he believes anti-psychotic drug treatment disturbs brain activation patterns.
Also, the majority of the earlier studies involving schizophrenia diagnosis were conducted in smaller samples and the results were unlikely to be replicated in larger samples.
The new 87% accuracy finding, which was published in the journal NPJ Schizophrenia, improves on a 2017 study on other machine learning models built for the same purpose, which returned a 74% accuracy result.
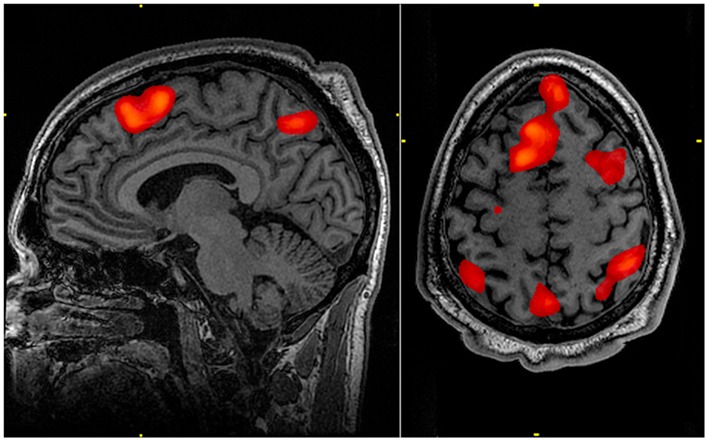
Advantages of using AI and machine learning for mental disorders
Advancements in AI technologies are bringing psychosis treatment to more people who need it.
It’s becoming clear among many industry commentators that AI for mental health could be a game changer.
Dr Kalmady described mental disorders – particularly in schizophrenia – as a “unique challenge” due to the complex nature of the patients’ illnesses, which can prevent them from communicating their symptoms effectively.
He identified the problem to be “objective measurements”, such as MRI and genetic testing, as these are inadequate in identifying clinical symptoms in schizophrenia.
Machine learning, on the other hand, uses thousands of engineered features to learn the combinations of their importance that lead to an optimal prediction.
Dr Kalmady said: “Although many individual variables in these measurements are known to be altered in schizophrenia at a group level compared to healthy individuals, they are typically neither specific nor sensitive enough to be used as a diagnostic tests.
“Artificial intelligence – more specifically machine learning – provides a way to ‘learn’ from the historical account of patients’ experience.”
Dr Kalmady believes such “learning” will enable the prediction on new unseen patients, which can be greatly beneficial when making key clinical decisions on the correct diagnosis and prescription of drugs.
He added: “This drives a practice of medicine that is truly ‘evidence-based’, which is particularly crucial for psychiatry.
“The next step in this line of research is to develop models for predictions on the course of the disease and responses to treatment.
“For instance, patients can greatly benefit from models that have learned to predict optimal treatment strategy that is individualised for them.”
Challenges of developing new schizophrenia tool
Dr Kalmady said the most difficult element of the EMPaSchiz research was to gather fMRI data from a large number of untreated patients with schizophrenia and healthy controls.
Another challenging factor was introducing “clever ways to reduce the dimensionality of fMRI data and extract relevant features that would be useful for the diagnostic task”.
Dr Kalmady said the team explored other research papers on existing methods to subdivide the brain into smaller chunks on the basis of varying physiological characteristics.
“We then developed an ensemble framework to jointly learn from the different perspectives of examining the brain signals,” he added.