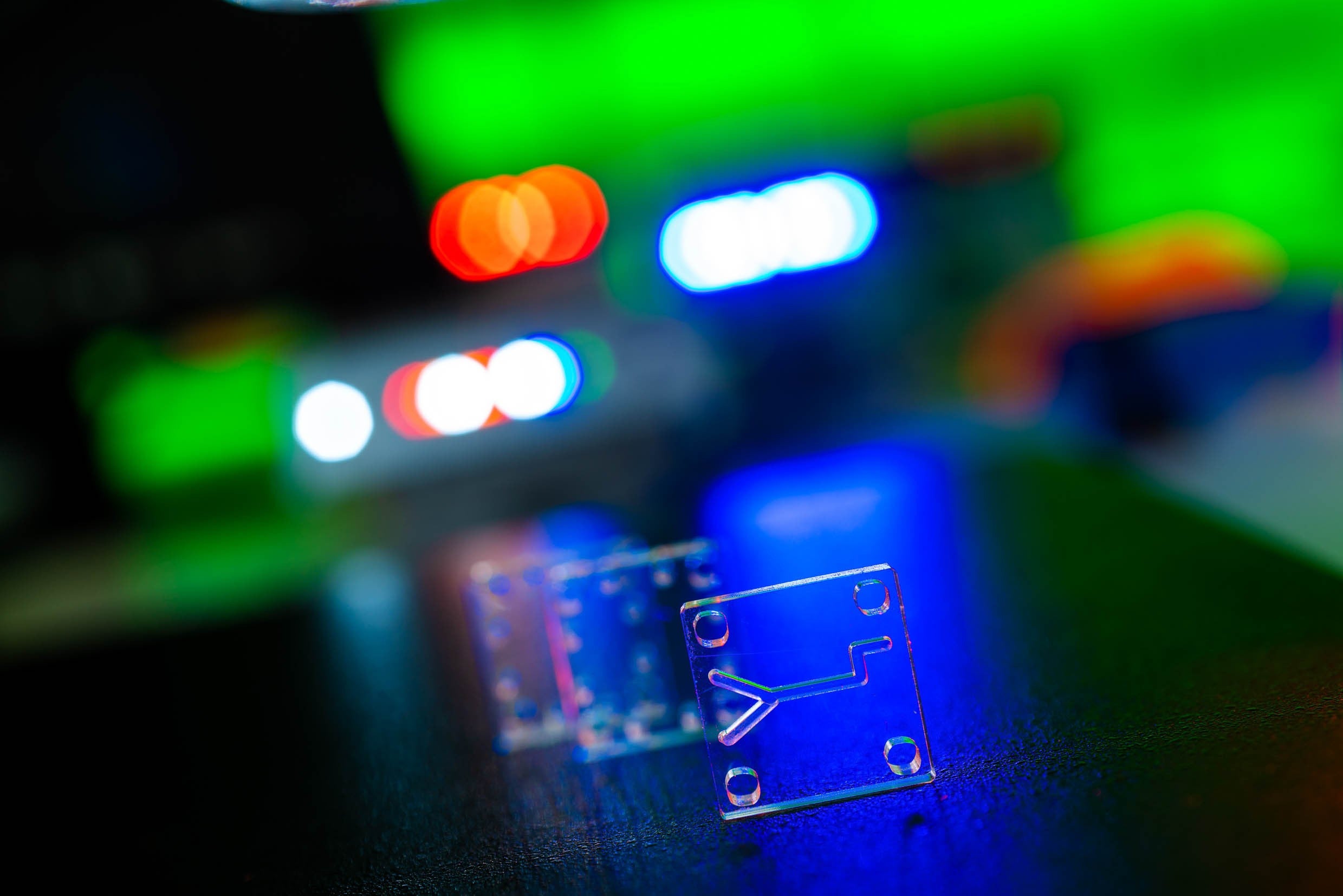
Microfluidics requires high levels of expertise, so simplifying through AI and machine learning would promote its broader use in healthcare and life sciences. Integrated machine learning and AI could allow people who are not experts in physics and engineering to run next-generation diagnostic tests, potentially improving treatment for many diseases, not least cancer. Jim Banks talks convergence with DeepCell co-founders Maddison Masaeli and Mahyar Salek, and Ali Lashkaripour, a research assistant in Boston University’s Cross-disciplinary Integration of Design Automation Research (CIDAR) group.
The ability to manipulate fluids at the micron scale has opened up a new realm of analytical processes, enabling relatively cheap devices to perform several functions simultaneously with tiny volumes of reagents.
In a device the size of a computer memory stick, it is now possible to simulate an entire human organ by lining microfluidic channels with organ-specific cells. Microfluidics provide an unprecedented wealth of data, but it has always required a high degree of technical skill.
Most obviously, microfluidics presents an engineering challenge, as it requires tiny amounts of liquid to pass through channels that might only span 10µm.
The technical expertise required has made microfluidic devices hard to replicate at scale, hence the drive to make them simpler to use and to add layers of digital technology to glean more insight from the data they can generate.
“We believe that leapfrog advances happen more often when technologies converge,” says Mahyar Salek, CTO, president and co-founder of Deepcell, which is launching a new platform for identifying and isolating cells based on morphological distinctions.
“The next big advance in microfluidics will be its combination with AI and other technologies.”
AI is perfectly suited to identifying patterns in large volumes of data and automating repetitive tasks. Machine learning (ML) enables a system to learn from data and improve its accuracy over time without being programmed to do so.
Both are part of the growing trend towards digital microfluidics (DMF) as a platform for lab-on-a-chip systems.
“I envision AI and ML greatly simplifying the design process, real-time control and data analysis of microfluidic devices,” says Ali Lashkaripour, a research assistant in Boston University’s Cross-disciplinary Integration of Design Automation Research (CIDAR) group. “Given enough data points, ML can take the engineering or the art out of any complex process.
“One of the most challenging and resource-intensive steps of developing microfluidic devices for diagnostics is coming up with a design that delivers the desired performance.Traditionally, this is achieved with expertise, ingenuity, and trial and error, which makes the design process expensive and challenging.”
“Helpfully, given enough data, AI algorithms can learn the relationships between design parameters and performance, and support optimal microfluidics design decisions. “That means we don’t need a microfluidic expert present at all times,” adds Lashkaripour.
“I have met many brilliant life-science researchers and almost all agree that microfluidics can significantly accelerate the discovery cycle. Researchers want microfluidics but don’t have the necessary expertise, resources or infrastructure. The high barrier to entry is significantly lowered if we have design automation tools.”
Speed up the design process through AI
Lashkaripour and CIDAR director Douglas Densmore have been working on automating the design of low-cost microfluidic droplet generators, recognising that droplet-based microfluidic devices hold immense potential as inexpensive alternatives to existing screening platforms in areas such as enzyme discovery and early cancer detection.
According to WHO, nearly 60% of deaths from breast cancer happen because of a lack of early detection programmes in countries with meagre resources.
“Microfluidics inherently comes with the promise of better data quality in terms of higher sensitivity and accuracy, and faster results with high throughput and quick reaction times,” Lashkaripour remarks.
“But the little secret is that developing these devices is extremely slow and expensive. ML models capable of predicting the performance of microfluidic devices can speed up the design process tremendously.”
CIDAR has developed a web-based tool, DAFD, that uses ML to predict performance and enable design automation of flow-focusing droplet generators. Lashkaripour hopes it will greatly reduce the need for microfluidic expertise and design iterations.
Design automation software could remove inefficiencies, both speeding up the design process and, crucially, making it cheaper.
Researchers who are often pursuing a moving target with changing performance requirements may no longer need to start the design process from scratch over and over again.
“Changing the geometry means fabricating another device in-house, which takes days and needs a cleanroom, or outsourcing it, which takes several weeks,” adds Lashkaripour.
“AI models coupled with automated search algorithms can rapidly suggest designs perfectly tailored to both performance requirements and user design constraints. If the goal is to minimise the sample volume while achieving a prescribed performance, AI models will rapidly test all possible designs in-silico and report the best one possible.”
Bring AI, ML and microfluidics into the commercil sphere
Deepcell, the company founded by Salek and CEO Maddison Masaeli, who invented its AI-based single-cell analysis and sorting technology, is among the first to take the combination of AI, ML and microfluidics into the commercial sphere.
The California-based company came out of stealth mode a few months ago and is gearing up to launch its platform, which identifies and isolates viable cells based on morphological distinctions for use in translational research, diagnostic testing and therapeutics.
The technology enables the delivery of intact and viable single cells with high precision and maintains cell viability for downstream characterisation. It can be used to isolate any type of cell, even those occurring at frequencies as low as one in a billion.
“Our focus is on morphology, which has only been used in a qualitative and descriptive way before,” says Masaeli. “There is a lot going on in a single cell. Our goal is to quantify it, to get morphology data at a massive scale so that we can take a big data approach to diagnosis.”
Deepcell’s mission is to enable novel biological insights at the single-cell level for improved diagnosis and treatment of disease. Microfluidics provides the single-cell data, which AI and ML then analyse for meaningful patterns.
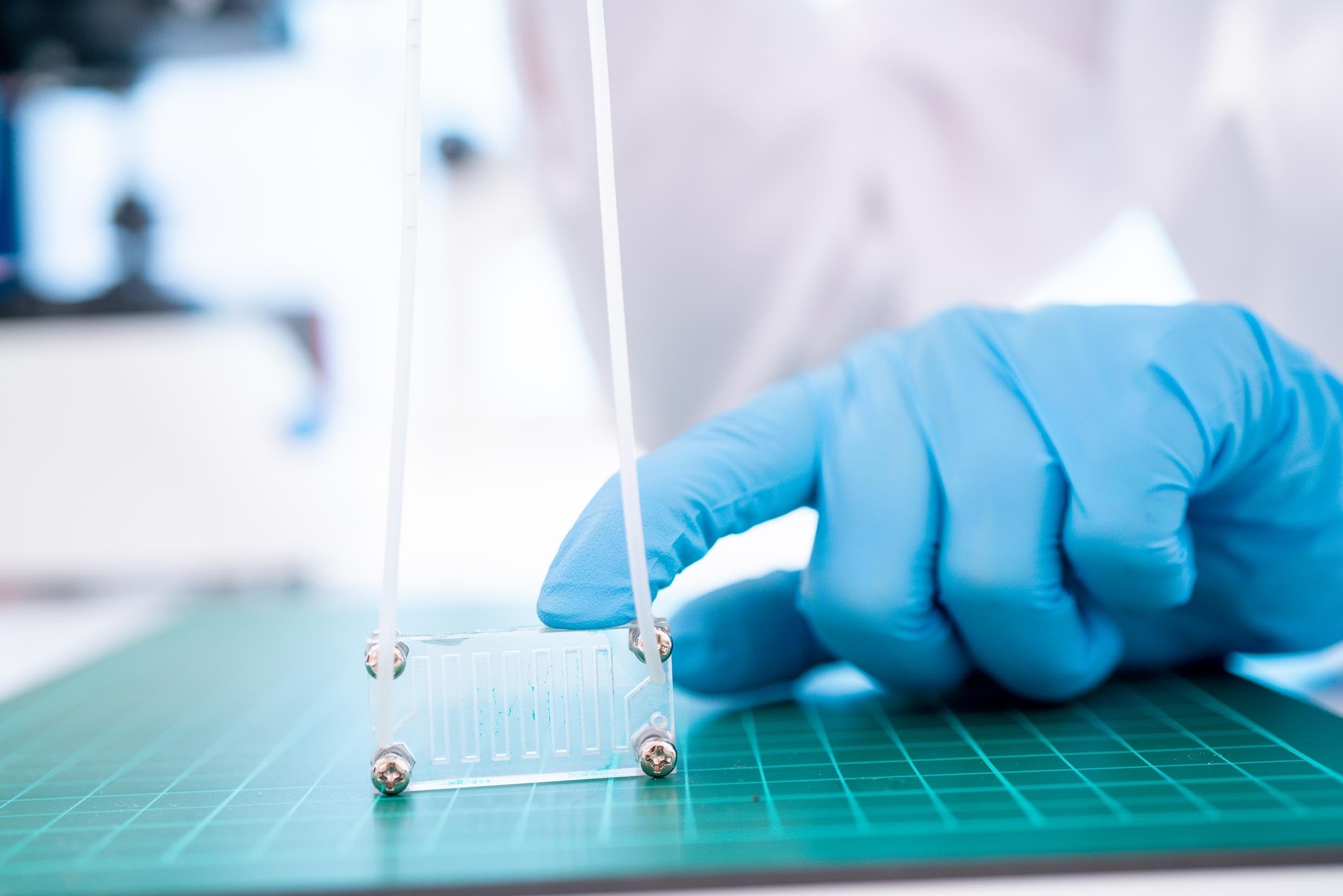
“Microfluidics provides clean data for our AI brain,” explains Masaeli. “We did a lot of processing and analysing to understand what data we need for the AI to identify different cell types. So, we can use ML to do the job of identifying cells on the basis of their morphology.
We can also use ML for the fabrication process, using non-human observation to see if the chip has been manufactured properly. Automating quality control is important because differences in microfluidics chips will affect accuracy.”
The platform automates the once-manual process of identifying cells by their morphology. The results can be used for profiling cells within the same tissue to understand variations in the expression of different genes and proteins, enriching them to study tumour microenvironments and even to improve the early detection of cancer by catching rare circulating tumour cells in body fluids.
“AI helps to translate the morphology into numbers,” says Salek. “Usually, that involves a lot of visual checks using a microscope, but the embedded AI now performs that task.
“Microfluidics is a very challenging field in terms of fabrication, repeatability and quality control. It is not for a non-expert. Part of our innovation is to streamline the process as much as possible to maximise stability and repeatability.”
A key feature of Deepcell’s platform is that cell classification is not limited by labels or markers. The AI is continuously learning to differentiate cells without inherent bias. For instance, people often label larger cells as malignant, which is not necessarily accurate.
The AI is trained on cells that have already been identified. No one is applying their own preconceptions of what a malignant cell should look like, which greatly improves accuracy.
Ultimately, the technology could have a huge impact on any therapeutic or research area in which cells are studied. In the future, it could conceivably enable the consolidation of many invasive tests into a single non-invasive one.
“There is a real hunger for a tool in this area,” says Masaeli. “Morphology is a very important phenotype that has often been left out, but people now want to try it on their hypotheses to achieve their translational and clinical goals.
For instance, non-invasive liquid biopsies could ultimately replace more barbaric procedures. It is all about identifying what is unusual and interesting using AI, which looks for patterns in data instead of someone looking at blood cells through a microscope.”
Other companies are also bringing AI-enabled microfluidics platforms to market. Nicoya, for example, has combined surface plasmon resonance (SPR) – an optical technique for detecting molecular interactions – with DMF.
Its Alto platform, which can direct and influence nanolitre droplets using electricity, is the first digital, high-throughput benchtop SPR system.
“Spatial transcriptomics developed by 10x Genomics is another great example of microfluidics and ML making it to the market,” says Lashkaripour.
“It works in conjunction with a droplet-based microfluidic platform to enable researchers to measure gene activity and map that activity to a location on the tissue sample. It can quickly lead to new insights for better understanding disease and developing novel diagnostics.”
Convergence of AI and microfluidics opens new doors for data
The convergence of AI and microfluidics is opening new doors every day. A group of electrical engineers, computer scientists and biomedical engineers at the University of California-Irvine recently reported the development of a new lab-on-a-chip using AI, microfluidics and nanoparticle inkjet printing that can improve the study of tumour heterogeneity, potentially enabling new approaches for reducing resistance to cancer therapies.
In its paper, the team showed that the platform allows for the precise characterisation of a variety of cancer cells, opening the door to a better understanding of tumour initiation, progression and metastasis, which could shape the development of better drug therapies.
“We are at the beginning of a scientific data boom, so ML will play an ever-increasing role in almost every field, including diagnostic tests,” believes Lashkaripour.
Where data is involved, AI and ML are rarely far behind. As companies like Deepcell make their platforms commercially available, the potential for microfluidics to become cheaper, more scalable and within the technical capability of non-experts will no doubt create a hotbed for innovation.
This article first appeared in Medical Device Developments Vol. 1 2021. The full publication can be viewed online here.