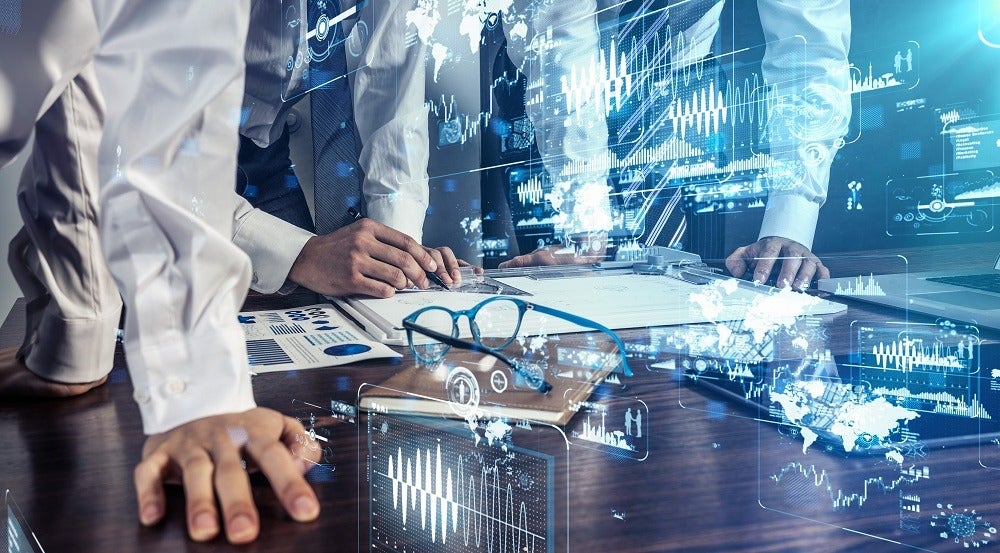
Since the discovery of AI and machine learning in the medical field, technology in the industry is creating AI-backed machines faster and better than ever, bringing new drugs and therapies to the market.
In a field where lives are at stake and every second matters, finding the key to build more accurate models for a wider range of conditions and diagnose at a better rate than doctors can is revolutionary.
Here we speak to Christian Hebenstreit, general manager and senior vice president of EMEA for Medidata, a company that uses AI and advanced analytics to help the clinical sciences.
He acknowledges the concerns regarding AI being a threat to replacing jobs as a hot topic due to its enhanced capabilities, but claims that most people aren’t aware of just how AI is being applied to actively save lives.
He said: “AI and machine learning have already made an impact in the life sciences industry, accelerating drug discovery and development.
“However, there’s still a significant opportunity to use these emerging technologies further to continue to improve efficiency, cut costs, extend research and most importantly deliver patient-centred experiences.”
The advantages of AI is noticeable during clinical trials and drug development stages – a procedure that took ten to 15 years and $2.6bn (£2bn) to complete as testing new drugs is a slow, expensive and manual process.
Therefore, big data and machine learning engineers are in high demand with companies turning to technology to accelerate the process.
Mr Hebenstreit said: “Big data, AI, remote monitoring and machine learning all have the power to catapult clinical trials into a ‘new era’ by helping to improve their efficiency and productivity, deliver novel outcomes, increase patient engagement and reduce patient burden.”
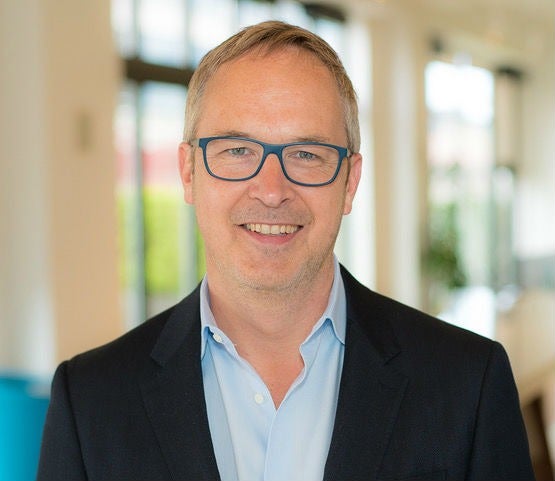
How AI and machine learning can speed up data production
From the moment of submission to the final standardisation of data in clinical trials, the steps required to filter through the vast quantities of complex datasets results in only a small number that can be used to advance further research.
Processing this huge amount of information can be a time-consuming task and impossible to carry out manually.
An example Mr Herbensteit illustrates is the gender, which can be described in 700 different ways, some of which will include manual errors such as typos and transcription errors.
Mr Hebenstreit said: “Even if clinical trial systems were straightforward and working optimally, standardising and preparing all the data for analysis manually would be a close to impossible task.
“AI can ingest millions of words of text, molecules, genomic sequences and images in minutes, aggregate the data and devise hypotheses beyond human ability.”
Moreover, such trials often requires steps to be repeated before proceeding to a new phase and can be extremely costly, which is why companies are introducing AI technologies such as machine learning and deep learning into the process.
Mr Herbenstreit added: “Algorithms can help automate this process of identifying discrepancies and standardising or querying unusual data – ultimately accelerating time-to-analysis and, in turn, time-to-market.”
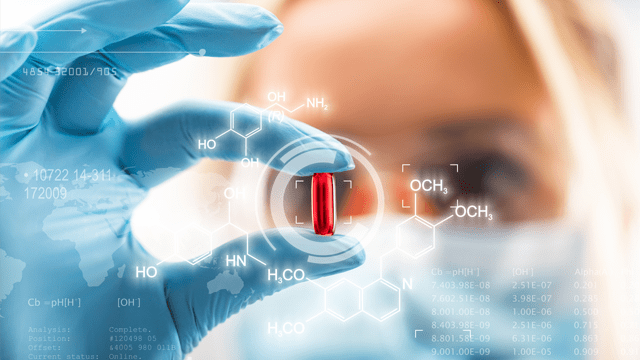
How AI and machine learning can eliminate risks and inefficiencies in clinical trials
Medical human errors cost society billions around the world. A recent estimate by Tech Policy Institute, a tech research and educational foundation, claims medical human errors in the US cost the country $17.8bn (£13.5bn) dollars in 2008.
With vast amounts of rich data, comes misleading results and errors that slows down the clinical trial process, and may even halt it altogether.
Mr Herbenstreit said: “There can be any number of reasons for why this happens – patient anomalies, a problem with the trial protocol or even fraud at a clinical research site.
“If drugs are submitted for approval based on unknowingly misleading data, it will be queried by regulatory authorities, adding months of extra work and cost for checking back through trial data for potential errors.
“Repeating elements of the trial and missing a critical window to bring a drug to market and a new treatment to patients.”
These disasters can be prevented by applying AI techniques, which promise to drastically reduce the number of mistakes made in the world of healthcare with their algorithms such as machine learning to predict these high-risk error situations.
AI is improving data quality with its capability to automatically identify areas of risk based on clinical study data anomalies before they severely affect trial process, “allowing organisers to change the trial protocol.”
Mr Herbenstreit said: “The beauty of machine learning and AI algorithms is that they can also learn and recognise points of commonality, anomalies amongst the discrepancies and help align data for accurate analysis.
“The technologies also ensure organisations and trials have a robust and clean dataset, which in turn accelerates drug approval processes and gets new, safe and tested treatments into the hands of patients faster.”
How to use AI and machine learning to gather genomic data faster for accurate results
The stream of health and genomic data available has drastically increased over the years.
Analysing the huge amount of data to extract the key biomarkers (a naturally occurring molecule, gene or characteristic) associated with a clinical response to a specific therapy needs a large team of scientific expertise and biomolecular experts.
However, Mr Herbeinstreit says, AI has the potential to extract relevant information and patterns from a patient’s medical records, compare it with ongoing trials and link it at the patient level to suggest matching studies accelerating biomarker discovery.
He said: “This in turn will speed up our understanding of diseases and possible treatments and allows the industry to begin to personalise medical treatments based on different genomics.”
According to PharmaTrend – a market and industry analytics platform – the interest in AI-driven solutions for early stage drug discovery is growing steadily among biopharma leaders with a projected market volume reaching $10bn (£7.6bn) by 2024 (for AI-based medical imaging, diagnostics, personal AI assistants, drug discovery and genomics).
Mr Herbenstreit added: “Machine learning tools have also enabled the discovery of novel biomarkers for Castleman disease, for example, including establishing new patient subgroups, based on previously unknown proteomic signatures.
“Such discoveries are helping to provide novel insights into treatment responses and potential new drug targets, highlighting the value of precision medicine made available through machine learning.”
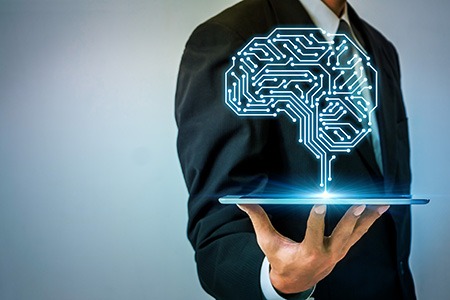
He also addressed how the vast amounts of data available to researchers is lowering the need for control samples as big data analysis techniques are making it increasingly possible to create synthetic controls digitally.
Mr Herbenstreit said: “This application of machine learning is also particularly pertinent during a time when recruiting patients to take part in clinical trials is becoming increasingly difficult.
“AI and machine learning are well-positioned to continue accelerating clinical trials, but this is provided advanced data infrastructures are in place, the right questions are asked, important goals are set and enough training data is provided.”
“If all these conditions are met, machine learning models working alongside AI have the potential to disrupt every stage of the clinical trial process – from biomarker discovery to monitoring adherence and data collection to the development of precision medicine .
“This will ultimately help to quickly close the gap between what patients have access to right now and what they will need in the future.”