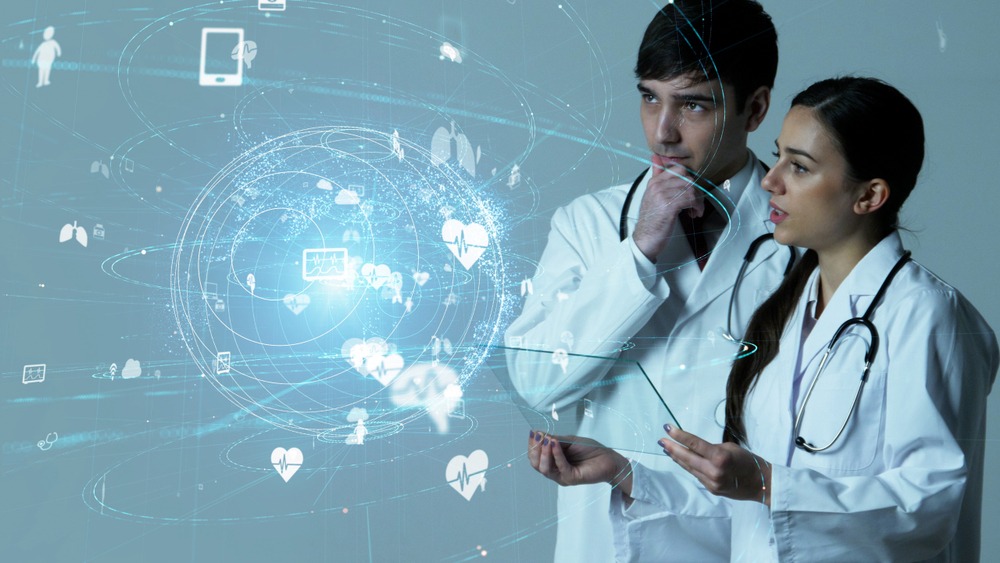
The healthcare industry benefits greatly from developments in AI due to the ability of algorithms to detect things on a larger scale and take certain actions faster than a human would.
As an area of study, there are many branches of AI that cover basic rule-based automation and machine-learning capabilities, all the way through to deep neural networks.
There’s still some trepidation among physicians and regulators about the use of AI in medicine, but it has made a dramatic impact on the way hospitals operate.
From scanning thousands of X-rays and detecting disease warning signs to assisting radiotherapists in precisely targeting tumours, we look at five ways AI is used in conjunction with medical devices in healthcare.
Five ways AI is used in healthcare:
1. Radiotherapy
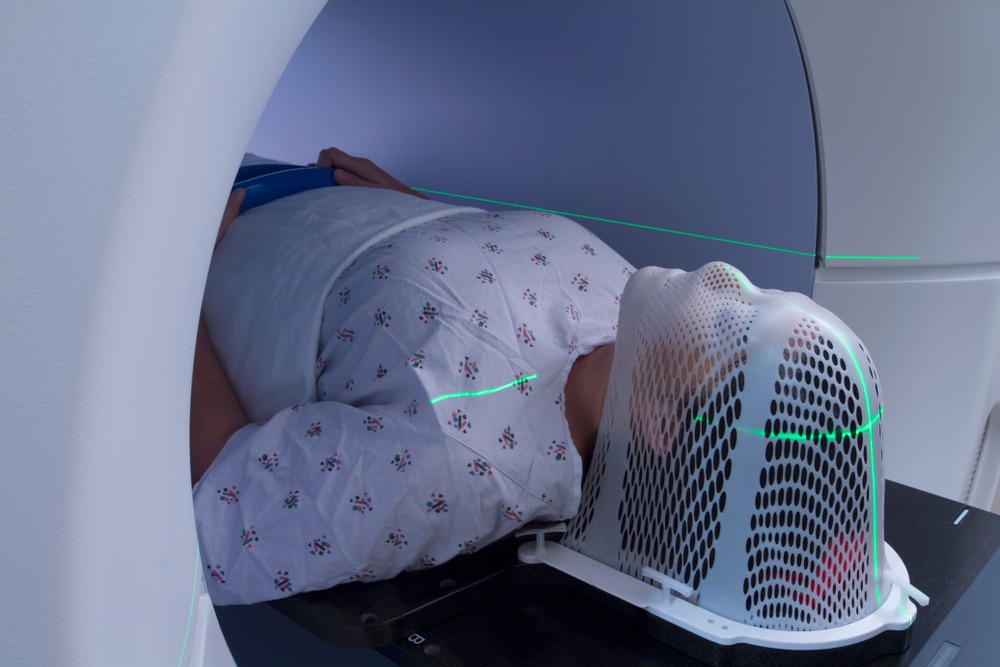
When the CT scan arrived and made the job of localising tumours easier, doctors still had to spend up to a week devising a treatment plan.
The use of a statistical analysis tool known as the Monte Carlo method allowed radiotherapists to run simulations based on knowledge about tumour density and beam characteristics in order to evaluate the risk of treatment on healthy tissues as well as calculate the dosage needed to destroy cancerous tissue.
But since then, the use of AI implemented at different stages has sped this process along even further, by using machine learning algorithms to detect and characterise tumour parameters and run simulations like this to output a treatment plan.
With a margin of error still in effect, these algorithms aren’t capable of achieving end-to-end treatment delivery without human input yet, but they do help guide radiotherapists and cut down the time it takes to diagnose, evaluate and deliver treatment.
2. Robotic surgery
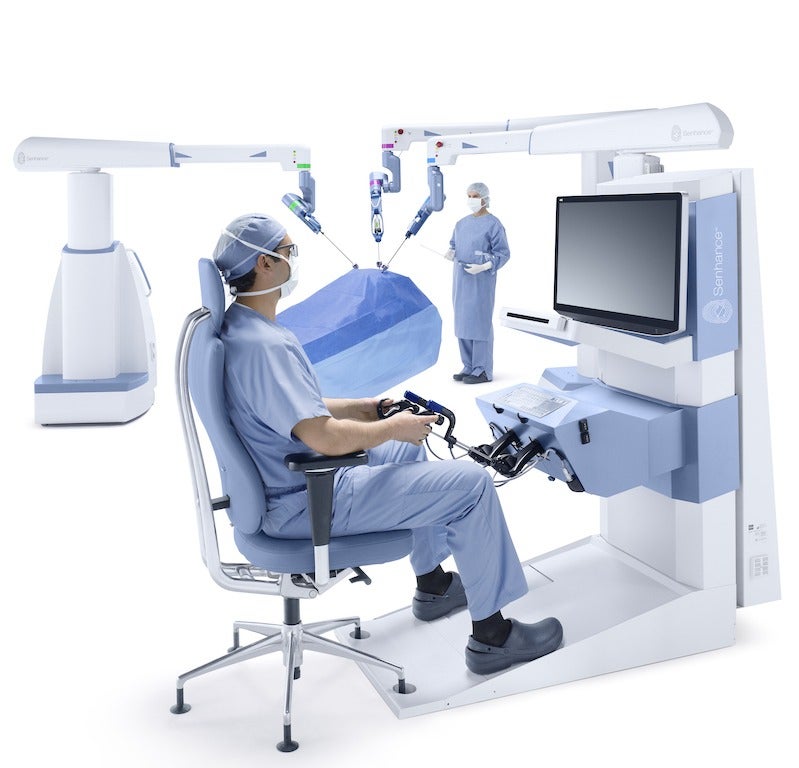
Robots have been in use to some extent in surgical theatres for decades, but with a host of options becoming available to hospitals, the next step to differentiate could be AI.
Asensus Surgical is pursuing just this by adding a navigation system to its robotic surgery system, named Senhance.
The Intelligent Surgical Unit received a CE mark in January after already holding an FDA clearance to market it back in March 2020.
The system uses machine-learning technology within Senhance consoles to record data from successful surgeries and, in the words of Asensus CEO Anthony Fernando, “learn from everywhere what good surgery looks like, and help deploy it anywhere.”
In practice, this will appear as physical cues for surgeons, and due to the nature of training a machine-learning system, it will apply more to routine, formulaic surgeries than those of a more complex nature that rely heavily on experience and skill.
3. Cardiovascular risk prediction
Coronary heart disease is the number one global killer, and one tell-tale sign of it developing is calcium deposits in the coronary arteries.
Typically, coronary artery calcification can be seen and measured with a heart-imaging test, like a CT scan – but radiologists aren’t abundant and nor is the equipment they use.
Researchers from Harvard Medical School affiliate Brigham and Women’s Hospital and the Massachusetts General Hospital’s (MGH) Cardiovascular Imaging Research Center (CIRC) teamed up to solve this issue by developing and evaluating a deep learning system that could lead to better detection of calcification, so that less patients fly under the radar.
The team validated the deep learning system in over 20,000 individuals and found the AI system’s results were “highly correlated” with the manual calcium scores from human experts.
Similar technology was touted during the Society of Cardiovascular Computed Tomography (SCCT) 2020 virtual meeting, and the Harvard researchers have open-sourced the algorithms used in the study, so the functionality could be in hospitals in the near future.
4. Breast cancer
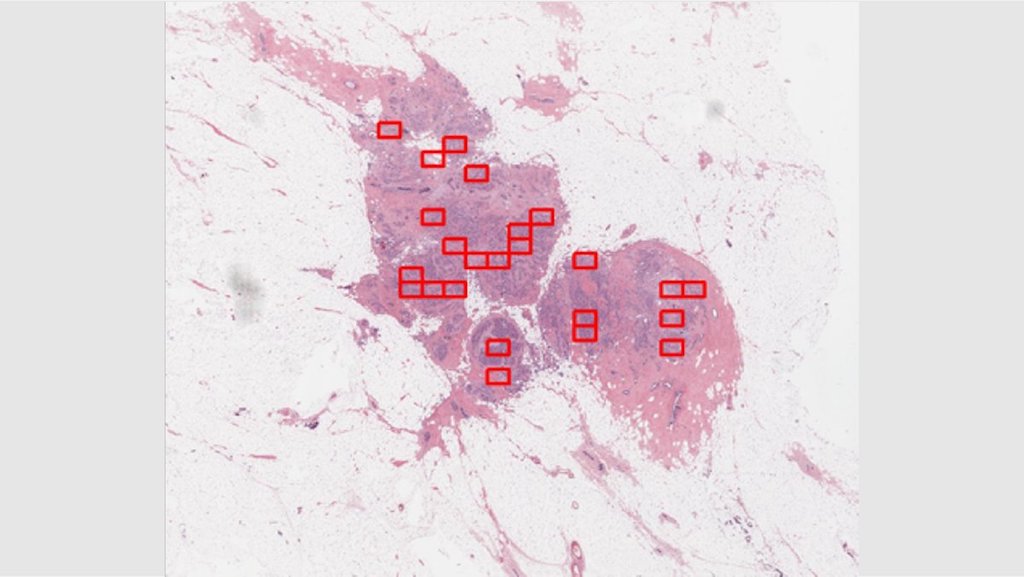
Automating the diagnosis of cancer could significantly improve the availability of testing and lead to more tumours being discovered earlier, rather than later, when treatment options can become less effective.
In November 2020, the FDA granted Breakthrough Device status to 4D Path’s precision oncology platform designed for diagnosing breast cancer.
The system uses AI to detect biomarkers for breast cancer in biopsy samples using what the firm terms “hidden data”, like heat signatures and cell-to-cell communication, in order to rule out certain mistakes that can and are made by human histopathologists.
The significance of this technology is that rather than sending off for a hematoxylin and eosin (H&E) stain as the first of many steps, an oncology department may be able to accurately diagnose and get a prognosis for breast cancers – and hopefully other cancers in time – without needing to run other tests.
4D Path is currently prepping its submission to for FDA clearance to market its AI technology to healthcare organisations.
5. Studying the affects of long Covid
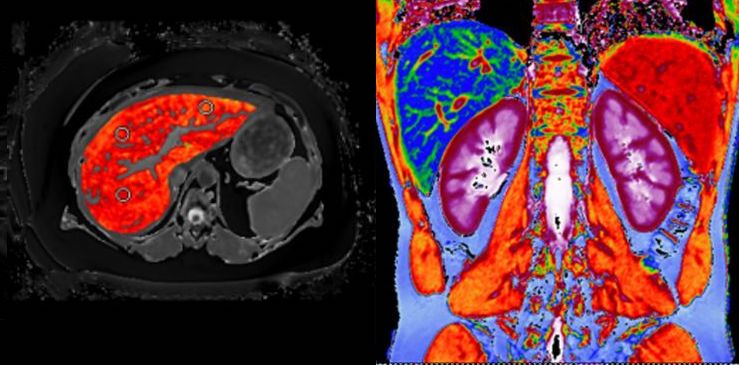
The deaths caused by Covid-19 have been the major story throughout the year 2020, but another aspect of the disease that continues to garner coverage is long Covid – particularly the way it can damage vital organs like the liver.
UK company Perspectum headed up the Coverscan study conducted in April using a combination of MRI scanners and AI to circumvent the need for invasive biopsy procedures and get a large sample size on which to draw conclusions.
In the case of a normal MRI scan, radiologists look at the image presented on screen and tell the patients what they find.
With Perspectum’s software, rather than taking an anatomy-driven approach looking for signs of disease in the liver, Perspectum uses computer vision to detect abnormalities at the cellular level that can indicate subtle signs of pathology.
The study found 70% of individuals in a young and low-risk population sample had impairment in one or more organs four months after initial symptoms.